What is so Exciting About The New Text-to-Image Transformer Model Muse by Google?
- Jan 2023
- 964
- 0
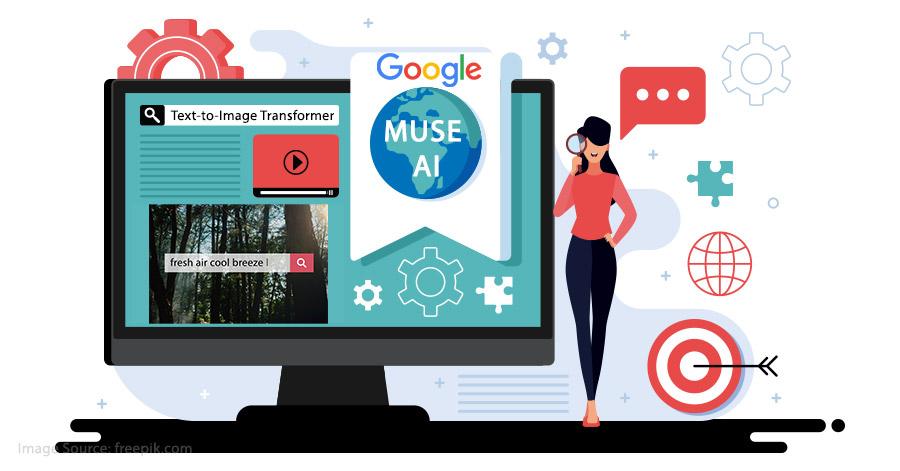
The Text To Image industry, with its deep learning-supported text-to-image models like as DALL-E-2, Stable Diffusion, and Midjourney, has been exciting.
However, with Google Muse AI, the most recent offering from the internet behemoth, the excitement has reached a new level indeed !!
The novel text-to-image transformer model utilises parallel decoding and a tiny, discrete latent space and promises to be faster than competing approaches.
According to its creators, Google Muse AI is capable of generating visuals at a cutting-edge level.
What are text-to-image transformers?
Text-to-image generation is a challenge in machine learning and natural language processing involving the creation of an image from a given text description. This might be a tough assignment since the model must comprehend the text's content and develop a picture to reflect it. There are several ways to address this issue, including the use of Generative Adversarial Networks (GANs) and Variational Autoencoders (VAEs). These models are trained on vast datasets of pictures and their related textual descriptions and are taught to create images that correlate to the written description. There are also more specialised models, such as DALL-E and AttnGAN, that are built exclusively for text-to-image creation. These models have achieved remarkable achievements in producing high-quality pictures from textual descriptions, and they have a broad variety of applications, including the generation of tailored product images and realistic avatar images.
What makes Google Muse AI special?
According to Google, Google Muse AI is an enhanced version of past text-to-image transformer models such as Imagen and DALL-E 2. Muse is trained on a masked modelling job in discrete token space utilising a pre-trained big language model's text embedding (LLM).
We present Muse, a text-to-image Transformer model that achieves state-of-the-art image generation performance while being significantly more efficient than diffusion or autoregressive models.
Google Muse AI team
Check this really informative video by Dr Alan D. Thompson that helps one understand more about Muse by Google AI
Do you KNOW?
Muse has been taught to recognise tokens inside arbitrarily veiled pictures. Muse promises to outperform pixel-space diffusion models such as Imagen and DALL-E 2 by using discrete tokens and requiring a lower sample size. The model generates a free zero-shot, mask-free edit by iteratively resampling image tokens based on a text prompt.
According to MUSE, compared to other models, Muse has quicker inference times.
Muse uses parallel decoding, but Parti and other autoregressive models do not. With a trained LLM, it is possible to comprehend language at a granular level, which translates to the production of high-quality pictures and the recognition of visual concepts like as objects, their spatial connections, posture, cardinality, etc. In addition, Muse allows inpainting, outpainting, and mask-free editing without the need to flip the model.
The Google Muse AI capabilities are really impressive
Muse is a quick, state-of-the-art text-to-image generating and editing system with a multitude of features:
Text-to-picture conversionIn response to textual inputs, Google Muse AI generates high-quality pictures fast (1.3s for 512512 resolution or 0.5s for 256256 resolution on TPUv4).
One of the major advantages of Muse is its Zero-shot, mask-free editing
Due to the repeated resampling of image tokens in response to a text query, the Google Muse AI model offers us with free zero-shot, mask-free editing.
When modifying a picture, mask-free editing enables the manipulation of many objects via a single text prompt.
Google Muse AI model details
The Google team used two distinct VQGAN tokenizer networks, one for low-resolution pictures and one for high-resolution images. Low-resolution ("base") and high-resolution ("superres") transformers are trained to predict masked tokens using the unmasked tokens and T5 text embeddings.
Comments
No comments yet.
Add Your Comment
Thank you, for commenting !!
Your comment is under moderation...
Keep reading blog post